TL;DR: DeepSeek's breakthrough reminds us how quickly AI capabilities and costs can shift. For companies building and deploying AI agents, this volatility creates unique pricing challenges. Static pricing models won't work when both technology and value delivery are constantly evolving. Companies need systematic approaches to price experimentation to stay competitive and capture fair value. Here's why this matters and how to adapt.
Why AI Agent Companies Must Rethink Pricing Now
DeepSeek's recent disruption of the AI model landscape carries important lessons for the AI agent ecosystem. When foundational AI capabilities can dramatically improve while costs drop, it impacts the entire value chain - including how we deploy and price AI agents. This raises critical questions: How do you price AI agents when their underlying capabilities are rapidly evolving? How do you capture value when each customer deployment might solve radically different problems?
Traditional SaaS pricing approaches - whether per-seat licenses or basic usage metrics - fall short in capturing the diverse ways AI agents create value. An agent reducing customer service workload by 40% delivers different value than one increasing sales conversion by 25%, yet many companies price them the same way. As agent capabilities grow, this misalignment between pricing and value will only increase.
A Real-World Pricing Challenge: AI Agents in Action
Consider this recent conversation between an AI Agent Company CEO and our CTO:
CEO: "Our agents are handling everything from customer support to sales assistance. Each deployment is unique, but we're stuck with one-size-fits-all pricing. How do we fix this?"
CTO: "The challenge is that agent value varies dramatically by use case. Support agents might save labor costs, while sales agents directly drive revenue. Your pricing needs to reflect these different value metrics."
CEO: "But how do we experiment with value-based pricing without disrupting our existing deployments?"
CTO: "This is where systematic price experimentation becomes crucial. Start by identifying key value metrics for each agent type. Then test different pricing models with new deployments while maintaining stable pricing for existing customers."
This exchange highlights the core challenge: AI agents often deliver value in ways that traditional pricing models can't capture. Without a framework for continuous price experimentation, companies risk either undervaluing their agents or losing ground to more adaptable competitors.
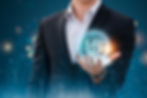
Why Traditional Pricing Models Fail for AI Agents
Rigidity:
Example: A subscription plan charging $500/month for an AI customer service agent is unfair to a startup using it 10 hours/month but a steal for an enterprise using it 1,000 hours.
Consequence: Startups churn; enterprises exploit underpricing.
Static Systems:
Legacy billing tools can’t process usage spikes or hybrid models. Example: An AI video editor charging per minute of output + premium features (e.g., 4K rendering) needs real-time rating.
Consequence: Manual invoicing delays or errors erode trust.
Misaligned Incentives:
Charging solely based on compute time fails to reflect real business value. Healthcare AI example: If one model diagnoses 100 patients in 1 hour with 95% accuracy while another takes 10 hours for 70% accuracy, pure usage-based pricing would actually charge more for the inferior solution.
Consequence: This ignores the true value delivered - faster, more accurate diagnoses. These misalignments become even more pronounced as AI agents grow more sophisticated and handle increasingly complex tasks.
All this complexity demands hybrid pricing models that combine different elements—usage, outcome, and feature-based pricing—to better align with customer expectations and value generation.
Key Considerations for Pricing AI Agents
Cost Dynamics:
AI agents incur variable costs: cloud compute ($0.10/hour), data licensing fees, and maintenance (e.g., retraining agent behaviors and updating knowledge bases monthly). Pricing must cover these while ensuring margins.
Value Metrics:
Align pricing with user outcomes, not effort. Example: A sales AI could charge per qualified lead generated, not per hour spent scraping data.
Customer Segmentation:
Tier pricing by use case:
SMBs: Prepaid, usage-based plans with caps (e.g., $0.10/API call, max $500/month).
Enterprises: Postpaid, outcome-based contracts (e.g., $10,000/month + 2% of revenue from AI-generated leads).
Market Agility:
AI markets evolve rapidly. Example: GPT-4’s launch slashed the value of older NLP models. Businesses must test pricing tiers (e.g., per-token vs. per-request) to stay competitive.
Best Practices for AI Agent Pricing
Prioritize Transparency:
Embed MTZ360's cost estimator widget into apps, letting users input hypothetical usage (e.g., “100 customer tickets/month”) to see projected fees.
Bundle Flexibility:
Combine models: Example: A “Pro Plan” with $300/month base + $0.05/transaction for fraud detection AI.
Align with Outcomes:
Charge for business impact: Example: A marketing AI charges 1% of attributed revenue from generated campaigns.
Experiment Continuously:
Run A/B tests on pricing pages: Example: Offer two plans to similar user cohorts—Plan A at $0.10/API call vs. Plan B at $100/month + $0.05/API call—to gauge elasticity.
How MTZ360 Addresses Generative & Agentic AI Monetization
Support flexible pricing models:
Subscriptions, pay-as-you-go, prepaid credits, or outcome-based. Example: An Email Agent is priced with a fixed monthly fee up to a delivery threshold, usage-based charges for excess volume, and optional fees for high-click-through campaigns.
Real-Time Rating Engine:
Accurately track compute consumption, user queries, and outcome-based metrics (like conversion rates or task completions) while instantly applying dynamic pricing rules, discounts, and usage-based adjustments.
Price Estimation:
Users simulate usage costs via a what-if calculator. Example: A marketing team models "What if we generate 500 AI-powered ad creatives?" and compares prepaid vs. postpaid pricing.
Price Experimentation Suite:
A/B Tests: Test $0.05/API call vs. $0.08/API call with 2% bonus credits.
Predictive Analytics: Use ML to forecast churn risk if prices increase by 10%.
Unified Billing:
Generate invoices in 100+ currencies, with prorations for mid-cycle upgrades. Example: A global Generative AI company scales from 10 to 10,000 API calls/day without billing chaos, reducing revenue leakage by 15%
AI agents are rewriting the rules of business—but monetizing them requires pricing models just as innovative. The future belongs to platforms that blend agility, transparency, and intelligence to capture fair value while driving customer success. Companies that master continuous price experimentation today will be better positioned to adapt to tomorrow's AI breakthroughs.
Ready to future-proof your AI Agent monetization?
Request a Demo: (https://www.monetize360.com/contact)
Learn More: (https://www.monetize360.com/mpower-enterprise)