The AI Disruption
How do you measure the value of AI in today’s software landscape? As AI becomes deeply embedded in CRM, ERP, analytics, and development workflows, traditional pricing models are struggling to keep up. The question isn’t just how we work with AI but how we align software costs with the benefits it creates.
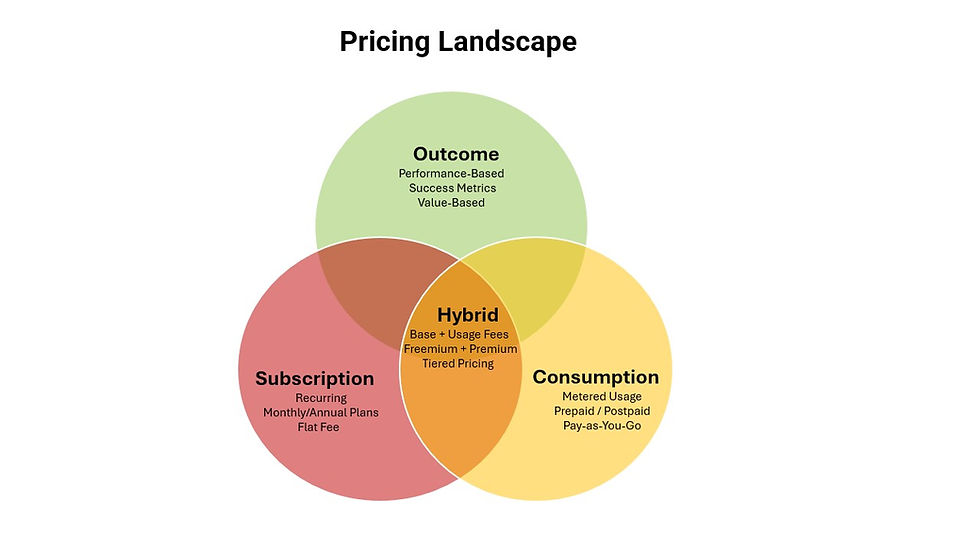
Limitations of Current Models
Are subscription models enough? They often miss the mark in capturing AI’s productivity gains.
What happens when AI takes on tasks traditionally done by multiple users? Per-seat pricing falls short.
Can fixed pricing reflect varying AI impact? Different businesses benefit from AI at different scales, making rigid pricing models impractical.
These challenges are driving the need for more adaptable, value-aligned pricing strategies.
Emerging Pricing Paradigms
Consumption-Based Pricing
This model charges customers based on actual software usage. It works well for cloud-based AI services, where usage metrics are easy to track.
Example:
- Microsoft Azure AI services charge by API calls or data processed. - Salesforce’s Agentforce product charges $2 per conversation, with discounts for high-volume businesses. This aligns pricing with usage but could pose cost challenges for smaller companies.
Outcome-Based Pricing
What if you only paid for the benefits your software delivers? That’s the idea behind outcome-based pricing—aligning costs with tangible results to ensure providers and customers benefit fairly.
Example:
- Zendesk is piloting this model for its AI-powered customer service tools, linking pricing to customer satisfaction or resolution times. The cost reflects key performance indicators, directly connecting fees with value delivered.
Hybrid Models
Some companies are blending traditional subscriptions with consumption or outcome-based components to offer more flexible pricing.
Example:
- Software providers may offer a base subscription with extra charges for AI-powered features or usage beyond set thresholds. This approach balances predictability with the benefits of usage-based models.
The Promise and Challenges of Outcome-Based Pricing
Outcome-based pricing isn't a new concept. In fact, it has been successfully implemented in various industries before the AI revolution:
1. Advertising: Pay-per-click (PPC) models revolutionized digital advertising, ensuring advertisers only pay when their ads generate user engagement.
2. Sales and Marketing: Many lead generation services charge based on qualified leads or actual conversions, aligning their compensation directly with client success.
3. Energy Efficiency: Some energy service companies price their solutions based on the actual energy savings achieved for their clients.
However, implementing it for AI solutions comes with challenges:
Defining Outcomes: Success can vary significantly between companies and industries.
Complex Measurement: AI solutions often interact with multiple systems, making it difficult to attribute specific results to one tool.
Risk Allocation: Providers and customers need to carefully negotiate who bears what risks.
Delayed Impact: Some AI benefits unfold over time, requiring long-term evaluation frameworks.
Integration Issues: Effectiveness hinges on smooth integration with existing processes, which isn't always straightforward.
Hallucination Risks: Paying only for genuine results is essential, which means robust outcome tracking is a must.
Despite these challenges, the flexibility and fairness of outcome-based pricing make it a compelling option as AI technologies advance and measurement capabilities improve.
Strategies to Overcome Implementation Challenges
Organizations exploring outcome-based pricing can follow these strategies to reduce risks and maximize success:
Set Clear Metrics: Define success metrics with transparency from the start.
Start with a Pilot Program: Test the pricing model on a small scale to fine-tune before full implementation.
Adopt Hybrid Models: Combine baseline fees with performance-based components to balance predictability and fairness.
Conduct Regular Reviews: Adjust pricing and metrics over time to reflect evolving outcomes.
Manage Risk with Caps: Use upper and lower fee limits to protect both parties.
Use Independent Auditors: Third-party validation helps ensure fair outcome measurement and reduces potential disputes.
A well-executed strategy can unlock the full potential of outcome-based pricing, providing both flexibility and value as AI technologies mature.
A Tale of Two Pricing Models: Code Generation Edition
Let’s look at AI-powered code generation through two pricing lenses:
Consumption-Based Model
- Pricing: Based on the number of lines of code or compute resources used.
- Tiers: Starter" (e.g., $X per 1,000 lines) to "Enterprise" (custom packages).
- Pros: Easy to measure; predictable costs.
- Cons: May not correlate directly with value delivered.
Outcome-Based Model
- Pricing: Based on improvements in development speed or code quality.
- Tiers: Linked to performance gains (e.g., 10%, 20%, or 30%+ improvements).
- Pros: Directly aligns with value creation.
- Cons: Requires robust tools like SonarQube or CodeClimate to measure outcomes effectively.
Choosing the Right Model
- Opt for consumption-based pricing if predictable costs or diverse use cases are priorities.
- Consider outcome-based pricing if you have clear, measurable KPIs and confidence in AI’s impact.
- Explore a hybrid model to combine the best of both worlds—starting with consumption-based fees and introducing outcome-based elements over time.
Embracing the Future of Software Pricing
AI is reshaping how we think about software—and pricing models must evolve with it. While outcome-based pricing offers the ideal alignment of cost and value, getting there will take time, with hybrid models acting as stepping stones.
The key to success lies in flexibility, transparency, and a willingness to experiment.
Are you ready to explore new pricing models for your AI workloads?
Join the Conversation
What’s your experience with these evolving pricing models?
How is your organization adapting to AI’s impact on software pricing?
Have you seen a shift toward usage-based models like Salesforce’s Agentforce in your industry?
Share your insights and help shape the future of pricing in enterprise software.
Turn Billing into a Strategic Advantage
Request a Demo: https://www.monetize360.com/contact
Learn More: (https://www.monetize360.com/mpower-enterprise)
Comments